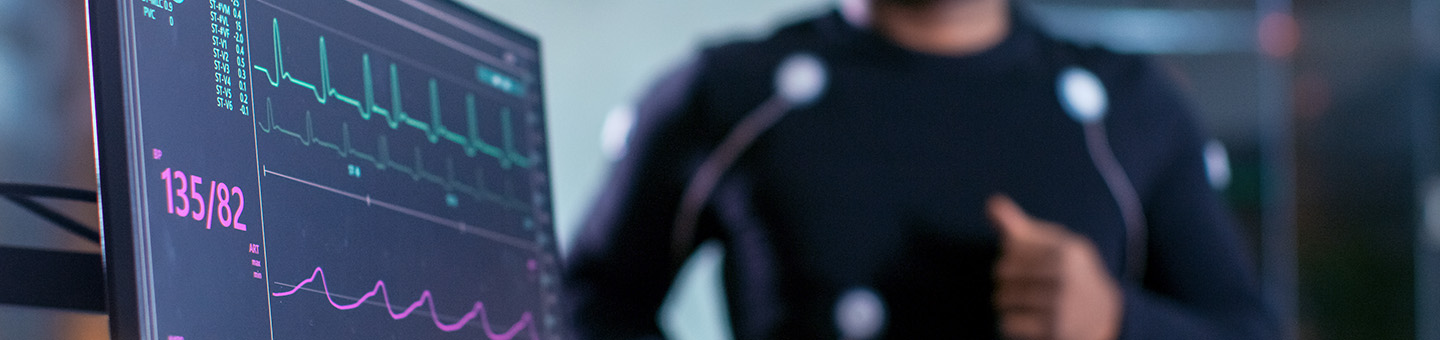
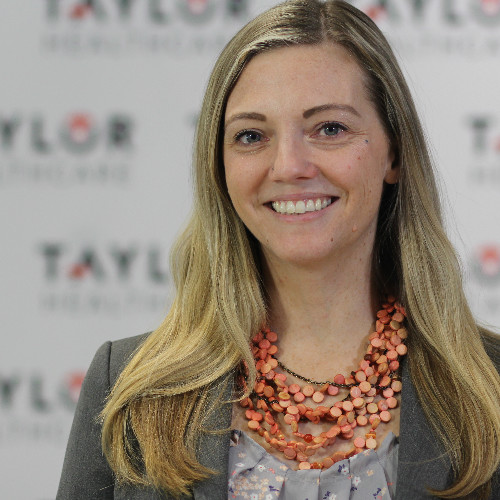
Chelsea Youngquist
Director of Marketing
Since the widespread adoption of Electronic Health Records (EHRs), hospitals are collecting more data than ever before Effectively analyzed and applied, this data can be used to increase efficiency of care, boost revenue, cut costs and improve health. However, as more information becomes available, some hospitals are having difficulty parsing what data is useful. As providers begin to understand the available data, curate it and ensure the people working with it can understand what is being measured, this undertaking will lead to improvements in several key areas.
1. Population Health
Data can be analyzed to examine the health and wellness of patient populations. With insights into socio-economics, genetics and patient history, providers can more thoroughly understand their patients and create better treatment plans. Data and analytics will allow providers better insight into population health epidemics such as heart disease, obesity, diabetes, and more recently, opioid overuse.
A number of potentially dangerous chronic conditions have emerged as major risk factors for the U.S. population, which has experienced an increasingly and extremely high obesity rate in recent years. Analytics will help to test and confirm health models for populations so providers can identify at-risk patients.
2. Improved Quality of Care and Patient Outcomes
Providers already know through their daily experience what is taking place. With a shift toward predictive analytics, healthcare providers can plan for what is most likely to happen in the future. This information helps predict which patient are potentially at-risk and create treatment plans to proactively address diseases.
With widespread EHR adoption and the progress in genome sequencing, physicians now have enough information to identify consistent patterns in symptoms and create accurate patient profiles.
As a result, there has been a boost in precision medicine. As David Shaywitz, chief medical officer at DNAnexus, a cloud-based data analysis and management platform for DNA sequence data, wrote in his article for Forbes: “The core premise … of precision medicine … is that the integration of genetic information, Electronic Medical Record data and rich dynamic phenotypic information will enable sophisticated patient segmentation, revealing biologically distinct subgroups and pointing the way to precisely targeted treatments.”
This will lead to the end of “one-size-fits-all” treatments in favor of personalized, more effective treatment. Instead of treating a patient for cancer, we will be able to define their specific symptoms of the disease, their individual condition and medical history — and even their genetic makeup — in order to tailor treatment and increase the chance of a positive outcome.
3. Reduced Costs
One of the many ways that predictive analytics help cut costs is by reducing the rate of hospital readmissions.
“The idea of predictive analytics comes in looking for relationships that are consistent with readmission that we would not have predicted or we did not understand before,” Mark Wolff, chief health analytics strategist for SAS Institute and an analytics software developer, says in a post on the Hewlett Packard Enterprise Enterprise.nxt blog. “Once we identify those relationships, we can set up protocols on how to deal with this type of patient and manage things to prevent readmission.”
UNC Healthcare, working to reduce readmissions, combined EHR information with geographic and socioeconomic data to better identify current patients at a high risk for readmission. UNC is working on ways to embed that intelligence into workflows to keep track of those high-risk patients to decrease the likelihood that health problems will occur. The predictive models they developed were more than 30 percent accurate at identifying at-risk patients.
4. Improved Patient Safety
According to the white paper How to Use Data to Improve Patient Safety, adverse events in healthcare are the third-leading cause of death in the U.S. — proving that while the industry may have done work and performed research to improve patient safety, it’s made little to no progress. In fact, patients today are experiencing 10 times the rate of preventable harm as they were in the 1990s. Without a data-driven, all-cause approach to patient safety, history will continue to repeat itself.
With initiatives around data-driven patient safety, health systems will have the tools they need to achieve better outcomes. Analytics will leverage integrated data and predictive analysis with machine learning to address organizational weakness and increase patient safety.